One of the most critical areas of development for financial institutions is risk management. Even though the average support center call is estimated to cost $4.00 in the US, adding up to a significant expense if a support center receives hundreds or thousands of calls per day, it does not compare to the cost and damage of various forms of fraud to banks, consumers, and merchants. The Ingenico Group estimates that merchants lose on average 1.5% of their annual revenue to fraud attacks, representing product and service losses, chargeback fees, and potential scheme programs. Meanwhile, identity theft and fraud cost consumers more than $16 billion.
Risk management practices have evolved over the decades of stumbling upon emerging and traditional risks in banking, changing the bank-FinTech narrative from competition towards a hybrid strategy. Estimates suggest that 8 out of 10 institutions foresee making strategic partnerships with P2P lenders, digital money transfer platforms, and numerous other firms that are reshaping the business of money. About 50% of the world’s financial services firms are planning to acquire FinTech startups in the next several years.
Heavy focus on adopting advanced technologies in risk management is also expected to impact the distribution of workforce and resource allocation. Estimates suggest that about 50% of staff today is dedicated to risk-related operational processes such as credit administration, while 15% focus on analytics. By 2025 – with the rise in technological advances – the numbers are expected to shift closer to 25% on operational processes and 40% on analytics.
When it comes to risk management in the financial services industry, the 430+ startups focusing on the applications of artificial intelligence and machine learning are in the spotlight. While it is not the largest segment out of close to 12,000 FinTech/RegTech/InsurTech startups operating worldwide, it is critical for bringing security into banking. AI/ML-powered risk management for financial institutions is embodied in advanced fraud prevention and AML solutions, as well as more accurate customer assessment.
Moody’s emphasizes that a machine learning model, unconstrained by some of the assumptions of classic statistical models, can yield much better insights that a human analyst could not infer from the data. At times, the prediction contrasts starkly with traditional models, the rating agency notes. According to Bloomberg, as financial services firms continue to improve their risk management processes and systems, many are putting AI to work to augment their current processes. The ability of ML models to analyze large amounts of data both financial and non-financial — with more granularity and deeper analysis – can improve analytical capabilities in risk management and compliance, helping analysts make more informed decisions at a securities level and across a broad-based, multi-asset portfolio.
AML/CFT
One of the ways financial institutions are required to control and report potential risks is through stress testing results submissions to the Fed, resulting in the adjustment of capital requirements for institutions. Stress testing submissions are vitally important for regulatory compliance.
Sharing one of the case studies, an AI company Ayasdi described its work with Citi, which consistently struggled to pass its annual stress test, failing two of the first three stress tests. The bank needed a way to rapidly create accurate, defensible models that would prove to the Federal Reserve that they could adequately forecast revenues and the capital reserve required to absorb losses under stressed economic conditions. The firm could not confidently defend the models they included in the filings they presented to the Federal Reserve. And to address the issue, Citi chose Ayasdi to supplement its capital planning process.
Just like financial institutions, regulatory bodies are equally interested in adopting advanced technologies for risk management. The Financial Stability Board (FSB) shares that some regulators use AI for fraud and AML/CFT detection. The Australian Securities and Investments Commission (ASIC), for example, has been exploring the quality of results and potential use of NLP technology to identify and extract entities of interest from evidentiary documents. ASIC is using NLP and other technology to visualize and explore the extracted entities and their relationships. ASIC has also used ML software to identify misleading marketing in a particular sub-sector, such as unlicensed accountants providing financial advice.
Another progressive regulatory body, the Monetary Authority of Singapore (MAS), explores AI/ML to analyze suspicious transactions. ML is being used to identify complex patterns and highlight the suspicious transactions that are potentially more serious and warrant closer investigation. Coupled with ML methods to analyze the granular data from transactions, client profiles, and a variety of unstructured data, ML is being explored to uncover non-linear relationships among different attributes and entities and to detect potentially complicated behavior patterns of money laundering and the financing of terrorism not directly observable through suspicious transactions filings from individual entities.
The Singapore-based OCBC Bank shared plans to use AI/ML as part of its efforts to reduce financial crimes. The bank intends to deploy these technologies to deal with the increasing scale and complexity of AML monitoring and increase the bank’s operational efficiency and accuracy in detecting suspicious transactions. OCBC Bank partnered with ThetaRay to use its AI solution to identify potentially suspicious transactions. The solution has reduced the volume of transactions reviewed by AML compliance analysts by 35% and increased the accuracy rate of identifying suspicious transactions by more than 4X. The bank plans to start an extended PoC and a pre-implementation phase. The algorithm will detect anomalies in transactional behavior by evaluating broad parameters such as products, customers, and risks, instead of looking at each transaction as a standalone. In the PoC stage, the technology was deployed to analyze one year’s worth of OCBC Bank’s corporate banking transaction data. The findings demonstrated that it decreased the number of alerts, which did not require further review by 35%. Read more.
Fraud, insider threats
Being one of the most forward-thinking institutions, Goldman Sachs has strong ties (as a customer and investor) with AI software provider Digital Reasoning, whose solution GS uses to track traders. The same startup has also launched a program with NASDAQ to use its AI technology to track trading data, communications, emails, chats, and even voice data to ferret out misconduct across the entire electronic stock exchange.
The CIO at HSBC, Darryl West, said the bank is using ML to identify patterns in the data to bring out what looks like nefarious activity within its customer base. The patterns that the bank identifies are then escalated to the agencies that the bank works with to track down the bad guys. The bank said that it is using Google Cloud ML capabilities for AML.
Another regulatory body, the US Securities and Exchange Commission (SEC), for example, leverages big data to develop text analytics and ML algorithms to detect possible fraud and misconduct. FSB shares that SEC staff uses ML to identify patterns in the text of SEC filings. These patterns can be compared to past examination outcomes with supervised learning to find risks in investment manager filings. The SEC staff claims these techniques to be 5X better than random at finding language that merits a referral to enforcement. In addition, unsupervised learning algorithms are used by SEC to identify unique or outlier reporting behaviors — including both topic modeling and tonality analysis.
Insider threats are consistently among the most significant sources of ongoing concern, which is why financial institutions invest in bringing automation into as many human-powered operations as possible. In 2015, for example, JPMorgan Chase made headlines when a former employee was arrested for stealing customer data and attempting to sell it to an undercover informant for tens of thousands of dollars. Barclays faced similar issues when criminals gained access to a USB flash drive with the personal details of more than 13,000 customers. In 2016, JPMorgan was set to spend a half-billion dollars on security.
To address the threat, at the end of January 2018, HDFC Bank had completed a pilot for AI-based Cyber Security Operations Centre (CSOC) to go live with it shortly after. The log data from CSOC is to be sent for processing on the AI solution, and it was done for around eight months on a cloud platform. The bank has close to 100,000 employees, and the AI solution is expected to help in monitoring insider threats.
The Financial Industry Regulatory Authority (FINRA) monitors roughly 50 billion market events a day, including stock orders, modifications, cancellations, and trades. The ML software FINRA is developing will look beyond 270 set patterns used to uncover potential rule violations and understand which situations truly warrant red flags.
Lloyds Banking Group has partnered with AI startup Pindrop to use its ML technology to detect fraudulent phone calls. Pindrop can identify 147 different features of a voice from a phone call or even a Skype call, which can help a person identify information such as the location that a caller is in, creating an audio fingerprint. Lloyds Banking Group will introduce the software across the Lloyds Bank, Halifax, and Bank of Scotland brands. Lloyds said the partnership with Pindrop will help it cut down call times as well as protect customers. Lloyds Banking Group also uses Google-backed AI to detect phone fraudsters.
Credit risk
Credit risk evaluation has evolved from classical linear, logit, and probit regressions to model credit risk to adopting AI/ML techniques to enhance credit risk management practices.
The opportunity to leverage machine intelligence to understand and repurpose consumer data found adopters in e-commerce. In their paper on the impact of AI and ML on risk management, Saqib Aziz and Michael Dowling of Rennes School of Business, France, share an example of ZestFinance, which partnered with Baidu to improve Baidu’s lending decisions in the Chinese market. Baidu was particularly interested in making small loan offers to retail customers buying products from their platform.
The authors explain that, unlike most developed countries, the risk with lending in the Chinese market is that less than 20% of people have credit profiles or credit ratings. Lending to people who have either ‘thin’ credit profiles or no credit profiles is inherently risky as there is no history to draw on to check the reliability of borrowers. ZestFinance (with permission) taps into the massive volume of information on members held by Baidu, such as their search or purchase histories, to help Baidu decide whether to lend. They use thousands of data points per customer and can still make lending decisions on new applications in seconds. A reported trial in 2017 of their system led to a 150% increase in total small-item lending by Baidu with no increase in credit losses in the space of just two months.
Aziz and Dowling note that the exact nature of how ZestFinance makes these decisions is not disclosed except under the broad umbrella of AI/ML. Essentially, what they will be using as a base is a core ML set of techniques around clustering and decision trees and possibly deep learning. Thus, a search history indicating accessing gambling websites, perhaps virtual private networks, or even types of search terms used, would cluster a potential borrower into a higher risk group. While on the other hand, a history of online spending that supports the applicant’s reported income in their application to borrow, or maybe even searches indicating career mobility upward, might cluster someone into a group of lower-risk borrowers.
Market risks and wealth management
Effective and gainful wealth management is directly tied to the ability to evaluate short and long-term market movements. One of the most interesting examples of a massive fund that brought AI into wealth management is Man Group, with about $96 billion under management at the end of 2017. Six years ago, engineers at Man Group built a system that evolved autonomously, finding moneymaking strategies humans had missed. The engineers, however, couldn’t explain why the AI was executing the trades it was making, which led to complete isolation of the system on a private cloud up until 2014 when Nick Granger decided to take it out of testing. Instead, Granger gave the AI system a small amount of money from a portfolio he was managing, gradually increasing the amount. At each step, the program was profitable.
Describing the timeline of the events, Bloomberg shares that by 2015, AI was contributing roughly half the profits in one of Man’s biggest funds, the AHL Dimension Program that managed $5.1 billion at the end of 2017, even though AI had control over only a tiny proportion of overall assets.
Initially, the concerned clients of Man Group started jumping on board. Four Man funds collectively managing $12.3 billion were incorporating AI at the end of 2017. Bloomberg shares that Man’s AUM surged about 77% between the beginning of 2014 and the end of 2017. AHL Dimension fund assets have more than quintupled. Man’s AHL Dimension fund, which started using ML in 2014, gained almost 15% in the three years through June 2017, almost double the industry average.
The impact of machine intelligence at the largest asset management firms cannot go without leaving a ripple on the market. Aziz and Dowling emphasize that one of the areas of focus within the market risk category of importance to large trading firms is understanding the impact of their trading on market pricing. One of the researchers they cite in their paper is Day (2017), who explores how large trading firms are using AI, particularly clustering techniques, avoiding the costs of trying to trade into and out of large positions in illiquid markets. He provides a quote from Capital Fund Management, one of the largest hedge funds in France with $11 billion under management, claiming that up to 2/3 of their profit from trades can be lost due to market impact costs. ML techniques significantly help with this issue by identifying connections between assets that are not easily observable and thus allow entering desired positions through a series of related assets rather than taking a large position in a single asset.
To learn about Prove’s identity solutions and how to accelerate revenue while mitigating fraud, schedule a demo today.

Keep reading

Discover tools and APIs for seamless, secure user onboarding that ensures a smooth experience while making accurate customer evaluations.
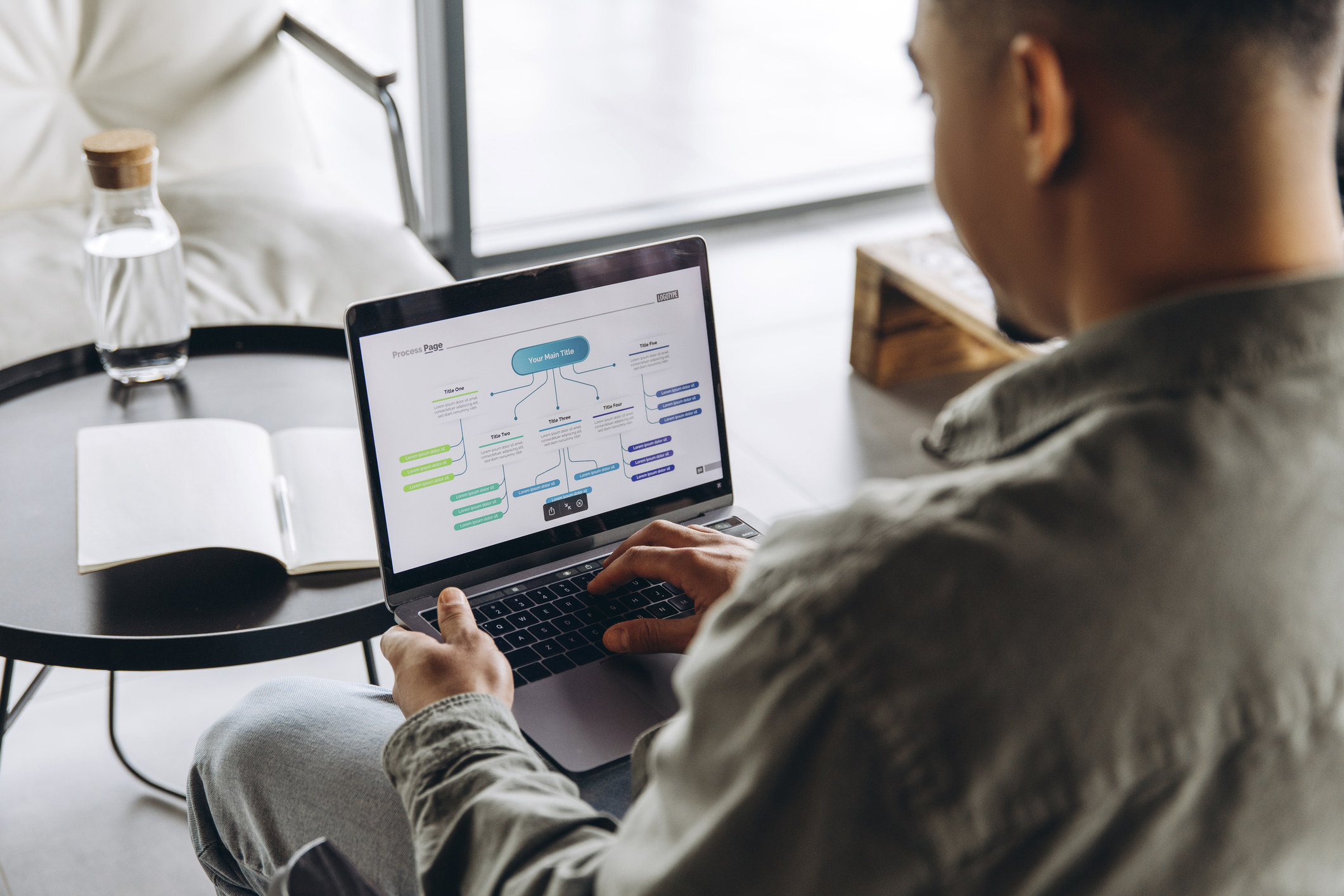
Learn how customer due diligence (CDD) verifies onboarded customers to keep your product secure.
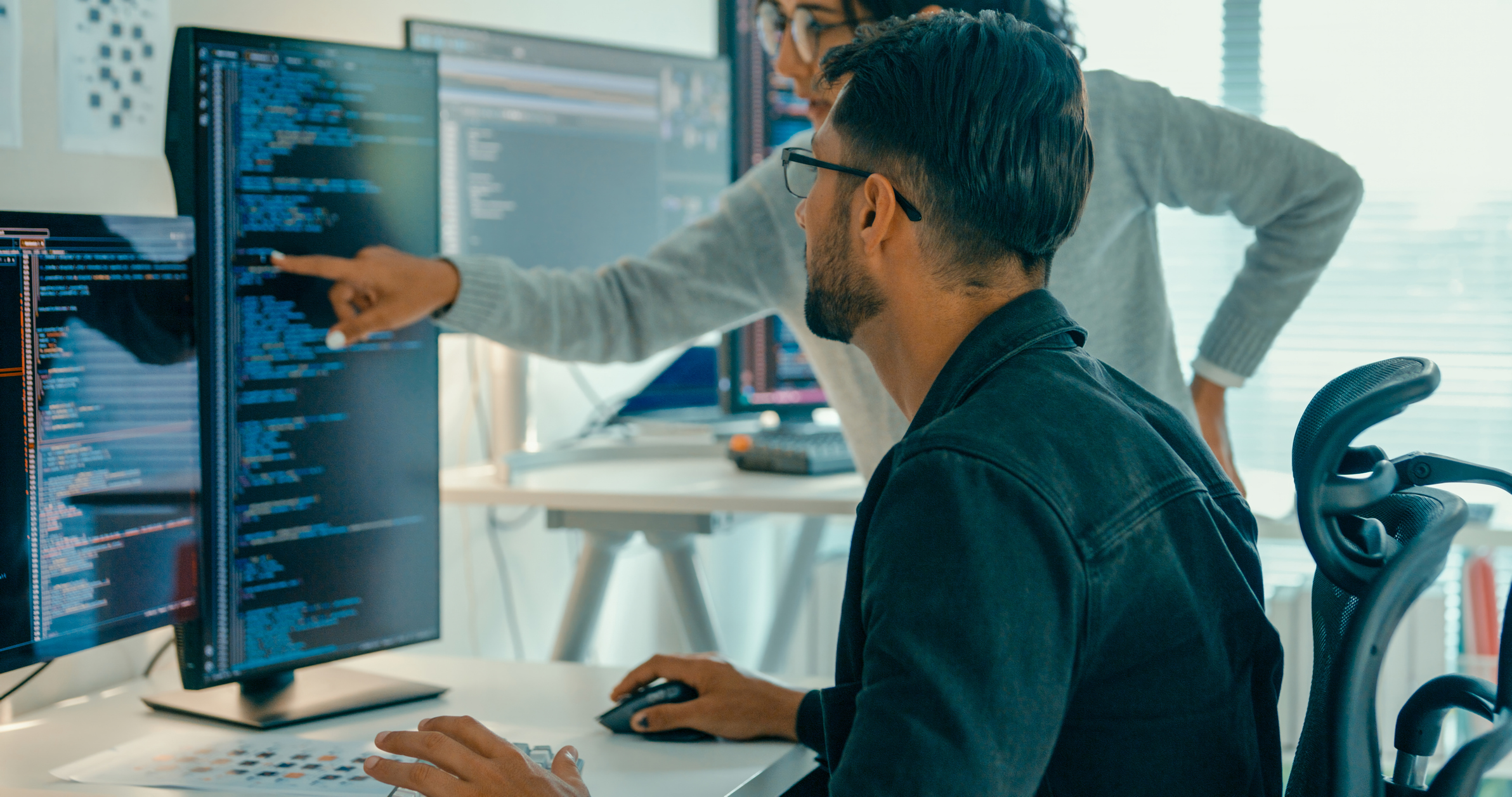
Learn about the new Developer Portal and how it can help developers implement identity verification.