Investing Intelligently: Applications of AI, ML & Big Data in Investment Management


Investment management is a broad field that could involve institutions or individuals; however, for this article, we took a look at the applications of AI/ML and Big Data in various domains or activities from the point of view of institutional investment managers:
Natural Language Processing (NLP): NLP is a subfield of computer science and artificial intelligence that focuses on programming computers to process and analyze human language. Vast volumes of non-numerical data are generated every year by companies worldwide in the form of annual reports and earnings calls. With growing applications of NLP in investment management, and coupled with ML techniques and data from non-traditional sources such as social media posts to identify trends, have helped Investment managers to better predict the impact on companies in specific regions and markets at large. Select applications of NLP in investment management are as follows:
- Detect change in sentiments: NLP can analyze news coverage, earnings calls, management speeches and detect a change in sentiments based on the tone of text or speech. E.g., Prattle (a US-based startup) uses NLP and ML techniques to analyze text and sentiments. Prattle provides predictive analytics and automated research reports on communications from approximately 3000 US public companies and 15 central Banks (including the Fed). Prattle assesses language used by central banks into quantitative sentiment data that projects policy outcomes. Prattle has predicted outcomes from the subtle language of central bankers with high accuracy.
- Topic Modelling: NLP can summarize a voluminous text into topics that humans can easily understand and can be used for analysis in statistical and machine learning applications. E.g., Portfolio managers at Goldman Sachs use NLP to analyze what topics the company management has covered in the earnings call this quarter versus the last quarter.
- Reporting: Computers require data for analysis, and people require language-based reporting to understand that analysis. NLP is capable of reducing this gap. E.g., Narrative Science (Chicago) is a company automating the writing of reports. The company’s product Quill creates applications that use natural language generation (a subset of NLP) software to assimilate data and then follows the same step that an analyst would take to write a report. Credit Suisse utilizes Quill to enhance its investment research products, HOLT. Quill has aided Credit Suisse HOLT to improve investment research coverage, quality, and consistency.
Portfolio Management: In the investment decision-making process, portfolio managers analyze plenty of capital market and economy-related data (e.g., GDP growth rate, inflation rate, historical returns on various asset classes). However, with the growth of Big Data in recent years, portfolio managers are using new AI/ML tools to analyze both the traditional and alternative sources of data to gain insights on security price movements and volatility to generate higher returns for their clients. The additional insights on security price movements also aid in managing the risk of the portfolio and deploying hedging strategies. An industry data firm, Barclay Hedge (not affiliated with Barclays Bank), conducted a poll in May 2018, which indicated that hedge fund managers are rapidly adopting machine learning. About 56% of the survey respondents (hedge fund professionals) said they use AI or machine learning in their investment process. Only 20% of the respondents had said the same in the previous year’s BarclayHedge Poll. According to a Financial Stability Board (FSB) report, among asset managers, machine learning is used widely by quant funds, and most of them are hedge funds. The report further states that it is hard to quantify precisely what proportion of quant funds use machine learning. To get a better insight into how some of the well-known players in the investment management industry are using Big Data and ML, an excerpt from Goldman Sachs Asset Management’s (GSAM) perspectives would be useful. (Please refer to more case studies at the end of the article).
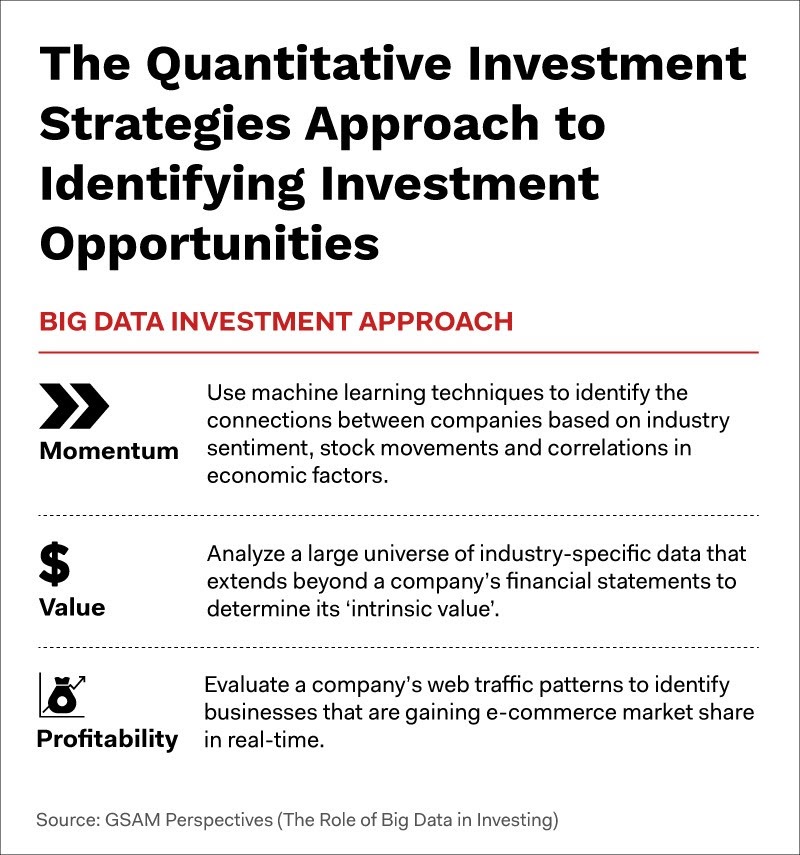
Algorithmic Trading: There is an increasing application of AI/ML in algorithmic trading to gain a competitive advantage. ML can accelerate and automate the search for effective algorithmic trading strategies, often a manual process. In addition, ML enables identification and adaptation to trends as they develop, rather than finding patterns based on historical data only. It also helps to find opportunities in many markets.
Turin Tech (a London-based startup) is an artificial intelligence company that provides an algorithmic trading platform offering automation based on machine learning models. The company provides an easy way for traders to develop and execute their trading ideas. The platform opens advanced trading to both retail investors and institutional investors. Recently, JP Morgan’s electronic equities trading division pioneered LOXM. LOXM is a self-learning trading algorithm with the ability to learn from the past and execute large complex equity trades without causing major market movements.
Robo-Advisors: Robo-advisor Wealthfront (US) has recently introduced an advice engine called Path that integrates third-party data with clients’ financial data and uses machine learning techniques to improve upon human judgment. Path advises clients on the future financial outcomes based on their current investments, risk tolerance, data from linked accounts, and assumptions compiled by Wealthfront. qplum (US) is another robo-advisor that combines AI/ML-based portfolios with human advice. qplum distinguishes itself by providing high-frequency trading techniques to optimize orders. It also provides automated risk management and dynamic asset allocation. qplum predicts that investors will become as comfortable with AI-based investing strategies as they are currently with mapping technology over the next decade.
Compliance Management: AI/ML-based solutions have a growing use in compliance management for regulators. In the US, the Financial Industry Regulatory Authority (FINRA) utilizes AI/ML-based solutions to identify potential cases of cross-asset manipulation. Cross-asset manipulation occurs when a trader holds an options position and attempts to move the underlying equity to change the equity price. Division of Economic and Risk Analysis (DERA) in the US Securities and Exchange Commission (SEC) uses machine learning to identify patterns in the text of SEC filings of investment advisors. These patterns are then combined with the outcomes of the past examination and then run through another ML algorithm that predicts the presence of risks in the investment advisor fillings.
Personalized financial recommendations to customers: Morgan Stanley recently launched its initiative called ‘Next Best Action’ to its more than 15,000 financial advisors. The technology behind the initiative works by examining communications with clients through emails or texts. The tool then applies machine learning to assess ideas that can be advised to the client. E.g., the tool could alert a financial advisor to send a message to a client when the firm has downgraded a stock in which he has a large position. Similarly, the tool brings several ideas to the advisor’s notice, and then the advisor decides which ideas are best suited for the client.
Case Studies: Leveraging AI/ML and Big Data for Investment Management
AI/ML techniques could have wider applications in the broader financial services industry (e.g., customer segmentation, robotic bankers, credit risk models, etc.). For now, we have attempted to highlight a few applications that are connected more directly with the process of investment management. Investment managers who can augment their investment decision-making process with the help of these technologies will have a competitive advantage over others.AI/ML techniques are still evolving, and it will likely lead to further innovation in the field and more application of these techniques in the investment management industry. As an American AI researcher, Eliezer Yudkowsky aptly puts it, “By far, the greatest danger of Artificial Intelligence is that people conclude too early that they understand it.” That hasn’t stopped companies in the FinServ space from actively seeking to leverage the power of these technologies to solve their problems. Here are a few interesting case studies of some companies that have applied AI, ML, and Big Data in their investment management processes:
- Blackrock: Blackrock, which recently established BlackRock Lab for Artificial Intelligence in Palo Alto to accelerate its efforts to bring the benefits of these technologies to the firms, uses Big Data extensively in investment decision-making. One of its investment products, called Advantage Range (diversified range of global and country funds), uses more than 100 signals into its investment process. E.g., investor blogs, atmospheric and oceanic trends, employee review sites, etc. In one of the case studies, a global fast-food chain with more than 14,000 store locations had remodeled store locations and changed its menu. Blackrock’s team (with expertise in Big Data and investments) models detecting foot traffic at restaurant locations began to reflect rising consumer activity. Macro models identified that the firm had locations in US regions where economic activity was picking up. Algorithms identified that the sentiment of sell-side analysts was becoming more positive. Blackrock’s team started investing in the company’s stock. After a few months, the company reported a better-than-expected quarterly earnings report, which increased in its stock price.
- JPMorgan: In another case study, JPMorgan traded retail stocks using buy-sell indicators provided by RS Metrics. RS Metrics (US) analyzes geospatial data from various sources, including satellites, drones, and airplanes. RS Metrics also provides signals, predictive analytics, and alerts, etc. With the help of 10 high-resolution satellites orbiting the Earth and imaging, the firm estimates retail traffic at some 50 retail channels. RS Metrics provided JPMorgan with their buy-sell indicators for a set of retail stocks together with their detailed signal construction methodology. JP Morgan’s strategy involved trading individual stocks based on the buy/sell indicators from traffic, and investment positions were taken and held until the next signal. JPMorgan traded S&P 500 stocks with a sufficiently long history of the signal, and the results were positive for most of them.
- Sigmoidal: The US-based Sigmoidal is a consulting firm specializing in applying AI to business. In one of its projects, Sigmoidal designed an intelligent asset allocation system that used deep learning and the Modern Portfolio Theory. The objective was to put into effect an investment strategy that could adapt to rapid changes in the market environment. The underlying AI model was tasked with predicting asset returns based on historical data. To achieve this, long short-term memory units (a generalization of a recurrent neural network) were implemented to achieve this. This model can learn long and complex temporal patterns in data. This helped Sigmoidal predict the asset’s future returns and the uncertainty of estimates using a technique called Variational Dropout. For robust predictions, Sigmoidal used lots of market data (currencies, indices, etc.), together with the historical returns of relevant assets. This resulted in over 400 variables used to make final predictions. Since many variables were correlated, Principal Component Analysis (PCA) was used, which decorrelates variables. Predictions of return and risk (uncertainty) for all assets were used as input to a Mean-Variance Optimization algorithm. Given the return’s predictions, this method determines a diverse allocation of assets at the lowest possible risk given the return’s predictions. Together, these models created an investment strategy that generated an 8% annualized return (significantly higher than any other benchmark strategy over two years).
Consider this: with industries investing aggressively in projects that utilize cognitive/AI software capabilities, estimates suggest that cognitive. Their AI spending will grow to $52.2 billion in 2021 and achieve a CAGR of 46.2% over the 2016–2021 period.
Based on what we see so far, it is evident that institutional investment houses are increasingly looking at new-age technologies more keenly than ever before. This is because they truly believe that the power of AI/ML and Big Data could aid them in research/analyses for better investment decisioning and also for improving productivity in operational activities.
To learn about Prove’s identity solutions and how to accelerate revenue while mitigating fraud, schedule a demo today.

Keep reading

This blog post explores the true meaning of online identity verification, debunking the myth that a simple badge guarantees authenticity and explaining the complex processes and tangible benefits of real ID verification for users.
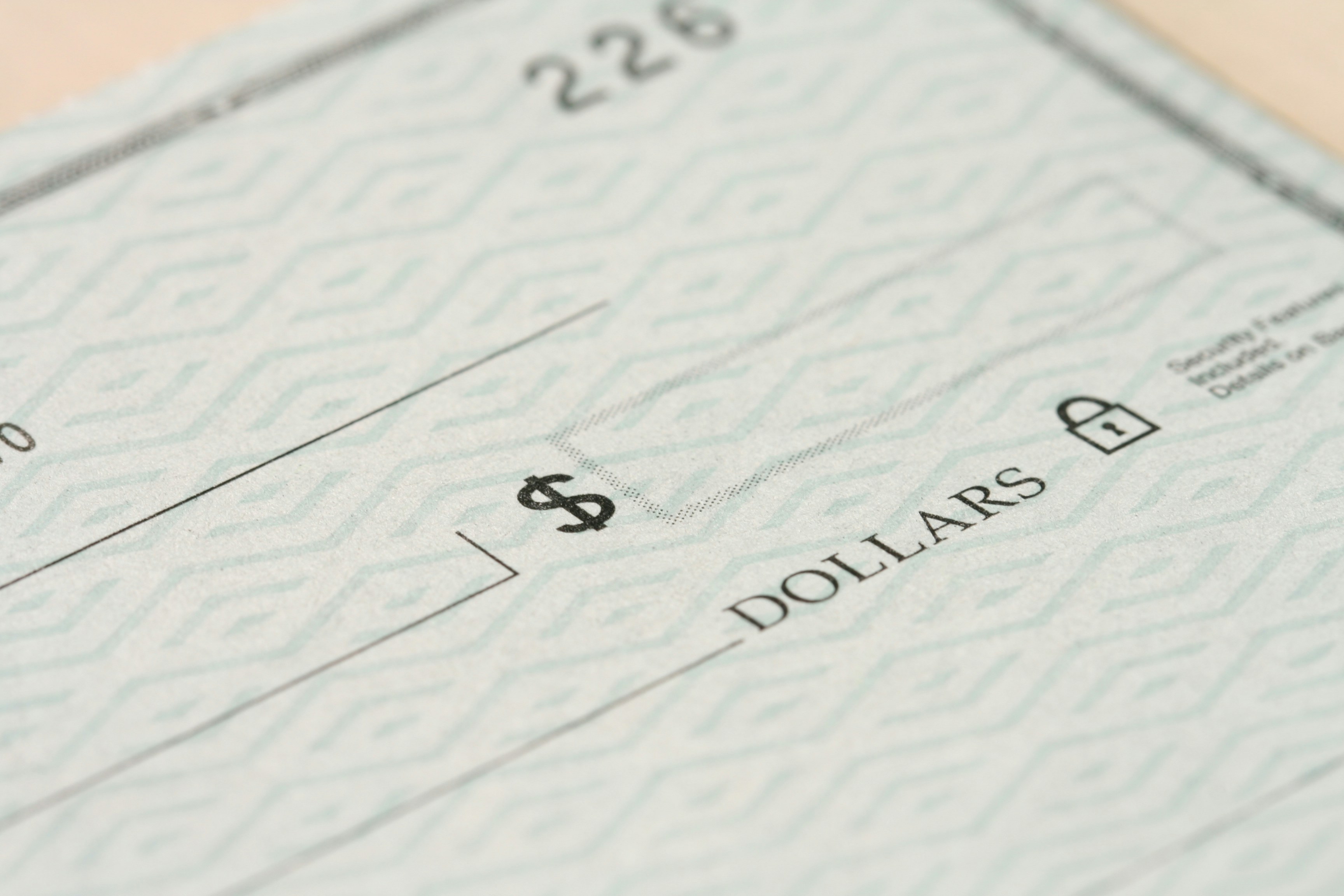
This blog post discusses the ongoing issue of check fraud in 2025, highlighting its impact on financial institutions and customers, and explores how technology-driven solutions like AI and automation can help prevent it.

In this article we showcase how Prove’s developer tools are revolutionized for identity verification. We examine their use cases, key benefits and industry applications.