Approximately 25% of consumers in the US are considered thin-file because they have fewer than five items in their traditional credit histories. About 7% are a no-hit (records other than the five items of the traditional framework), and about 9% are completely invisible—they have no record of credit. Globally, about 1.7 billion adults remain unbanked—without an account at a financial institution or through a mobile money provider.
One of the problems with extending financial access is the lens through which the formal financial system assesses one’s creditworthiness. Lenses vary in different countries, but one is constant—existing frameworks have not been able to effectively expand the addressable market. Financial technologies, however, have a role to play in changing the very framework.
With the abundance of digitized behavioral data available to those companies today—social media, search, mobile money, bills, shopping history, rent, etc.,—anyone can lend. Facebook alone targets ads based on 98 data points on every user. The number of FinTech startups in the alternative credit scoring space varies from several hundred to hundreds of thousands. The hallmarks of one’s lifestyle imprinted in continuous data flow are increasingly becoming vital in innovative ways to assess how trustworthy one is. The limitations in existing underwriting processes have been widely highlighted with professionals emphasizing the following:
- Credit scores provide limited insight into a consumer’s true financial position—they don’t provide the whole picture. With about a third of US consumers having a FICO score under 670, most traditional lenders would not offer loans to individuals with scores that low. But many of these people are creditworthy borrowers. FICO’s data doesn’t help in the assessment of whether they would repay loans—something that new data sources can help to predict more accurately.
- Copies of bank statements are susceptible to fraud. With banks encouraging their customers to move to online bank statements, obtaining paper copies for application processes can introduce delays and lead to high drop-out rates.
- Self-reported income is also susceptible to fraud and does not reflect any change in circumstances that may impact the ability to make repayments in the future.
But when 1.7 billion people don’t have a history with the formal financial system and existing frameworks are largely exclusive, how can institutions reach a balance of accurate risk assessment and continuous inclusive development? According to Experian, in the consumer financial marketplace, alternative credit data refers to information used to evaluate creditworthiness that is not usually part of a traditional credit report. Some examples the agency brings up:
- Rental payments
- Mobile phone payments
- Cable TV payments
- Bank account information, such as deposits, withdrawals, or transfers
- Small-dollar loans
Other types of alternative data might relate to things less closely tied to a person’s financial conduct, like that person’s education or occupation. As with anything alternative to established frameworks, there are always challenges to overcome, generations of releases to test for new frameworks to take hold. With alternative sources of data, there are still significant shortcomings to overcome.
1. Security, accuracy, fraud
Alternative data is subject to significant alteration of opportunities as it can be affected by fraudulent activities. With phone usage records, for example, the problem of cramming can potentially lead to downgraded scoring if a person is unable to detect malicious charges by various services leading to increased billing.
With utility payments, seasonal spikes in energy consumption in some regions can make a significant difference in the financial standing of low-income groups of the population, playing their scores in disadvantageous ways. Security breaches of utility providers aren’t off the table either. At the end of 2017, for example, the sensitive account and personal information of as many of 52,000 customers of The United Illuminating Co., Connecticut Natural Gas, and Southern Connecticut have been exposed to potential identity thieves as a result of a security breach by a third-party vendor.
According to the CFPB, though traditional data can also be inaccurate, certain types of alternative data may be more prone to errors if the standards governing the data are different or weaker than those governing traditional data. Consumers might not be able to access or view some types of alternative data. This could prevent consumers from finding and correcting any inaccuracies.
Consumers themselves are often enabling exposure to sensitive information—the culture of oversharing and blind trust in social media-bred a new type of fraud. Nearly 20% of social media accounts associated with 10 major global brands are fraudulent. With every fifth social media account being fraudulent, the use of social media data for creditworthiness assessment requires appropriate standards to ensure data integrity.
2. Data privacy and data ownership
The ability to build a comprehensive portrait based on alternative sources of data requires access to those sources, which itself raises other classes of issues—data privacy and ownership. A wide variety of continuous large-scale fraud cases and cybersecurity breaches have illustrated the significance of possible security risks.
“As the data sets that financial institutions utilize expand beyond traditional consumer credit histories, data privacy will become a growing concern, as will data ownership and whether or not the consumer has any say over how these data are used and shared or whether they can review it for accuracy.” – Lael Brainard, a member of the US Federal Reserve’s Board of Governors where she serves as Chair of the Committees on Financial Stability, Federal Reserve Bank Affairs, Consumer & Community Affairs, and Payments, Clearing & Settlements
The CFPB also notes that some alternative data may not be related to a person’s own financial conduct, and the use of these data could make it more difficult for people to improve their credit standing. Alternative credit factors may also be harder to explain to people seeking credit.
3. Lack of transparency
Although imperfect, traditional creditworthiness assessment frameworks can rarely be criticized for the lack of transparency. The FICO score, for example, has been molded into components, and every scored individual can understand what criteria affect their score. The use of thousands of data points by private companies to build alternative frameworks is rarely transparent for consumers to understand the weight of a wide variety of pieces of information put together to create a score.
“It may not always be readily apparent to consumers, or even to regulators, what specific information is utilized by certain alternative credit scoring systems, how such use impacts a consumer’s ability to secure a loan or its pricing, and what behavioral changes consumers might take to improve their credit access and pricing.” – Lael Brainard
With web search history, for example, there is a possibility of negative outcomes the use of such sort of alternative data can have, as Casey Oppenheim, Co-founder & CEO of Disconnect, fairly points out. Oppenheim emphasizes that nobody understands the long-term impact of this data collection, and there is no telling what happens to decades of one’s search history and how it shapes someone’s future. The readily available lifetime of search history traps one in the outcome of the past ‘mistakes’—unless the judging algorithm does not rule out the data from 20 years ago when a person's search would indicate inclinations towards activities incompatible with the idea of a trustworthy person. And it’s not just the problem of being stuck in an obsolete portrait due to massively affecting the end result data from 10 years ago, but also the problem of fairness and transparency.
4. Informed gaming
On the opposite side of the lack of transparency is informed gaming, when consumers are aware of the variables having weight on their risk profile and find ways to alter those variables in their favor.
Some professionals believe that assessment frameworks involving alternative sources of data may lead to system gaming practices in the form of segregation, for example. Once it has been figured out that certain connections on social media may negatively affect creditworthiness, people may start deleting negatively affecting connections.
“What we are finding is that yes, indeed, individuals [could game the system], if they could know somehow that you are a financially responsible person and I am financially responsible, and we all need to show that we are good individuals to the company on social media so that we can be considered worthy of a loan. We find that individuals will have some incentives to drop their friends or at least make the information, the connection of having a friendship with [certain people] less visible.
“What that could do over-time is [cause] some sort of fragmentation in social networks. Good types, people who are more financially responsible, have incentives to drop the bad types. That’s true for the bad types as well. They have an incentive to be connected to a higher number of [financially responsible people]. And they have an incentive to be connected to a smaller number of bad types. That’s going to result in, over time, a segmentation or fragmentation of the markets.” – The Surprising Ways that Social Media Can Be Used for Credit Scoring, Wharton
5. Discriminatory scoring
Back in 2016, Lael Brainard shared, “Non-traditional data, such as the level of education and social media usage, may not necessarily have a broadly agreed upon or empirically established nexus with creditworthiness and may be correlated with characteristics protected by fair lending laws. To the extent that the use of this type of data could result in unfairly disadvantaging some groups of consumers, it requires careful review to ensure legal compliance.”
While non-traditional data may have the potential to help evaluate consumers who lack credit histories, some data may raise consumer protection concerns.
The CFPB also emphasized potential discriminatory implications in the use of alternative data and modeling techniques. The authority shares that, for example, using alternative data that involves categories protected under federal, state, or local fair lending laws may be overt discrimination. In addition, certain alternative data variables might serve as proxies for certain groups protected by anti-discrimination laws, such as a variable indicating a subscription to a magazine exclusively devoted to coverage of women’s health issues. And the use of other alternative data might cause a disproportionately negative impact on a prohibited basis that does not meet a legitimate business need, or that could be reasonably achieved by means that are less disparate in their impact.
“Machine learning algorithms that sift through vast amounts of data could unearth variables, or clusters of variables, that predict the consumer’s likelihood of default (or other relevant outcomes) but are also highly correlated with race, ethnicity, sex, or some other basis protected by law. Such correlations are not per se discriminatory but may raise fair lending risks. The use of alternative data and modeling techniques could potentially lead to a disparate impact on the part of a well-intentioned lender as
well as allow ill-meaning lenders to intentionally discriminate and hide it behind a curtain of programming code.” – CFPB
6. Unintended consequences
With alternative data, financial institutions move into uncharted waters with a lack of experience in understanding the long-term impact of such an approach and appropriate history-proven algorithms for assessing that data. The new approach may not be consistent with its overall business strategy and risk tolerance of the formal financial system, including regulators.
“Banks collaborating with FinTech firms must control for the risks associated with the associated new products, services, and third-party relationships. When incorporating innovation that is consistent with a bank’s goals and risk tolerance, bankers will need to consider which model of engagement is most appropriate in light of their business model and risk-management infrastructure, manage any outsourced relationships consistent with supervisory expectations, ensure that regulatory compliance considerations are included in the development of new products and services, and have strong fallback plans in place to limit the risks associated with products and partners that may not survive.” – Lael Brainard
More importantly, we are mostly unaware of unintended consequences coming from highly varying lifestyles and circumstances of millions of lives.
In its request for information regarding the use of alternative data and modeling techniques in the credit process, CFPB emphasizes certain groups or behaviors could be penalized or rewarded in ways that are difficult to predict. For example, members of the military may frequently move, and the perceived lack of housing stability or continuity may give a false impression of overall instability. Or negative inferences could potentially be drawn about consumers who are not found in the alternative data source being used by the lender.
“Foreseeable or otherwise, using alternative data and modeling techniques could also cause potentially undesirable results. For example, using some alternative data, especially data about a trait or attribute that is beyond a consumer’s control to change, even if not illegal to use, could harden barriers to economic and social mobility, particularly for those currently out of the financial mainstream.” – The CFPB
Conclusion
The long-term social and economic consequences of developing and deploying systems that integrate vast granular information about every individual are not clear yet, but they certainly contain ever-important security concerns.
Bringing together personal data from numerous sources is an extremely curious exercise, but also a magnet for a more dangerous fraud than ever—in case an individual’s record is compromised, the whole life of that person gets affected, not just a particular part of it.
However, with proper security measures, such systems will have a strong transformative power over societies and enforcement of behavioral norms as they would directly connect everyday behavior with long-term prosperity, financial and otherwise.
Examples of FinTech companies mixing alternative sources of data into scoring frameworks
- Lenddo: Lenddo creates credit scores using social media.
- Facebook: In 2015, Facebook secured a patent that allows creditors to assess your creditworthiness based on the credit rating of the people in your social network.
- Neo: Neo can offer lower-interest-rate loans after considering the quality of connections on a LinkedIn profile.
- ZestFinance founder (former Google CIO): “People who type only in lowercase or uppercase letters are more likely to be deadbeats (all other things equal).” ZAML considers 70,000 signals and feeds them into 10 separate underwriting models.
- Kreditech: Looks at information from social networks, which is voluntarily shared by applicants. What type of friends do you have? Do you live in one place and always party in another? It looks at 8,000 indicators.
- Kabbage: Social media data is being used to help approve loans for small businesses.
- DemystData: Integrates social along with telecommunications information and corporate data to create the risk profile associated with an individual or small business.
- Happy Mango (credit score based on cash flow management): Allows users to submit testimonials about their character—positive feedback provides 10% of a score, which is on a scale of 0 to 100.
- LendUp: Looks at social media activity to ensure that factual data provided on the online application matches what can be inferred from Facebook and Twitter.
- TrustingSocial: Credit scoring 2.0. Its engine extracts tens of thousands of data points from social networks (Facebook, Twitter, LinkedIn, Weibo). It tracks hundreds of signals to detect any anomalies in behavior, network, and interaction patterns.
- China’s credit rating for everything: Traditional, social, and online inputs to the total score. The online score includes interactions with other internet users, ‘reliability’ of information posted or reposted online, and shopping habits.
- FriendlyScore: FriendlyScore uses over 820 variables contained within existing online social profiles to generate a holistic view of a person’s creditworthiness.
- Credit Sudhaar (credit advisory, Arun Ramamurthy, Co-Founder): Alternative sources of data, including social media, are an important part of creditworthiness assessment. It is only through the use of psychometric tests, social media data, and other unconventional sources of information can companies and banks identify the intention of a potential borrower.
To learn about Prove’s identity solutions and how to accelerate revenue while mitigating fraud, schedule a demo today.

Keep reading
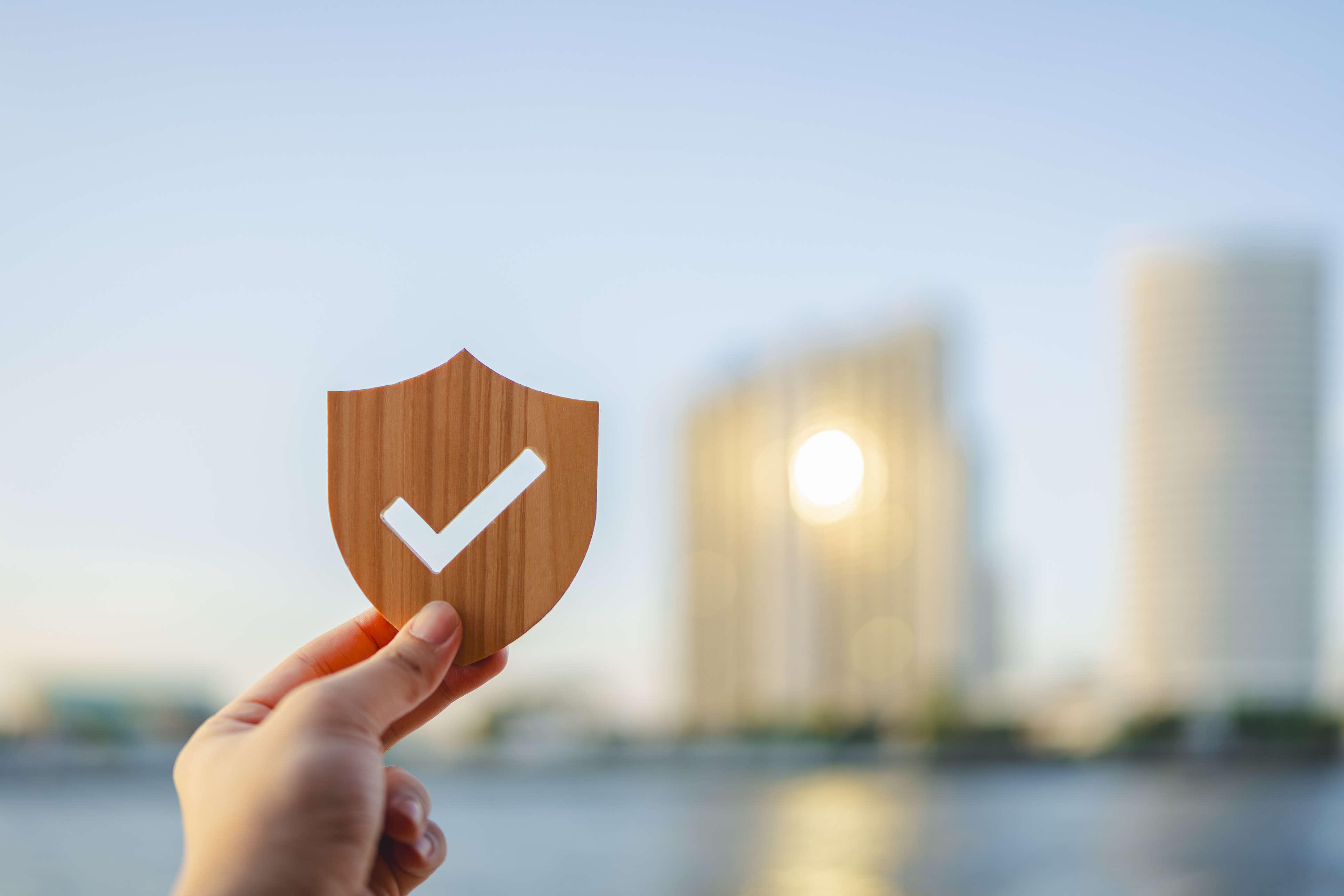
Learn why the increasing frequency of data breaches, coupled with fraudsters' ability to exploit numerous accounts, necessitates comprehensive data hygiene practices to protect personal information.

This blog post explores the true meaning of online identity verification, debunking the myth that a simple badge guarantees authenticity and explaining the complex processes and tangible benefits of real ID verification for users.
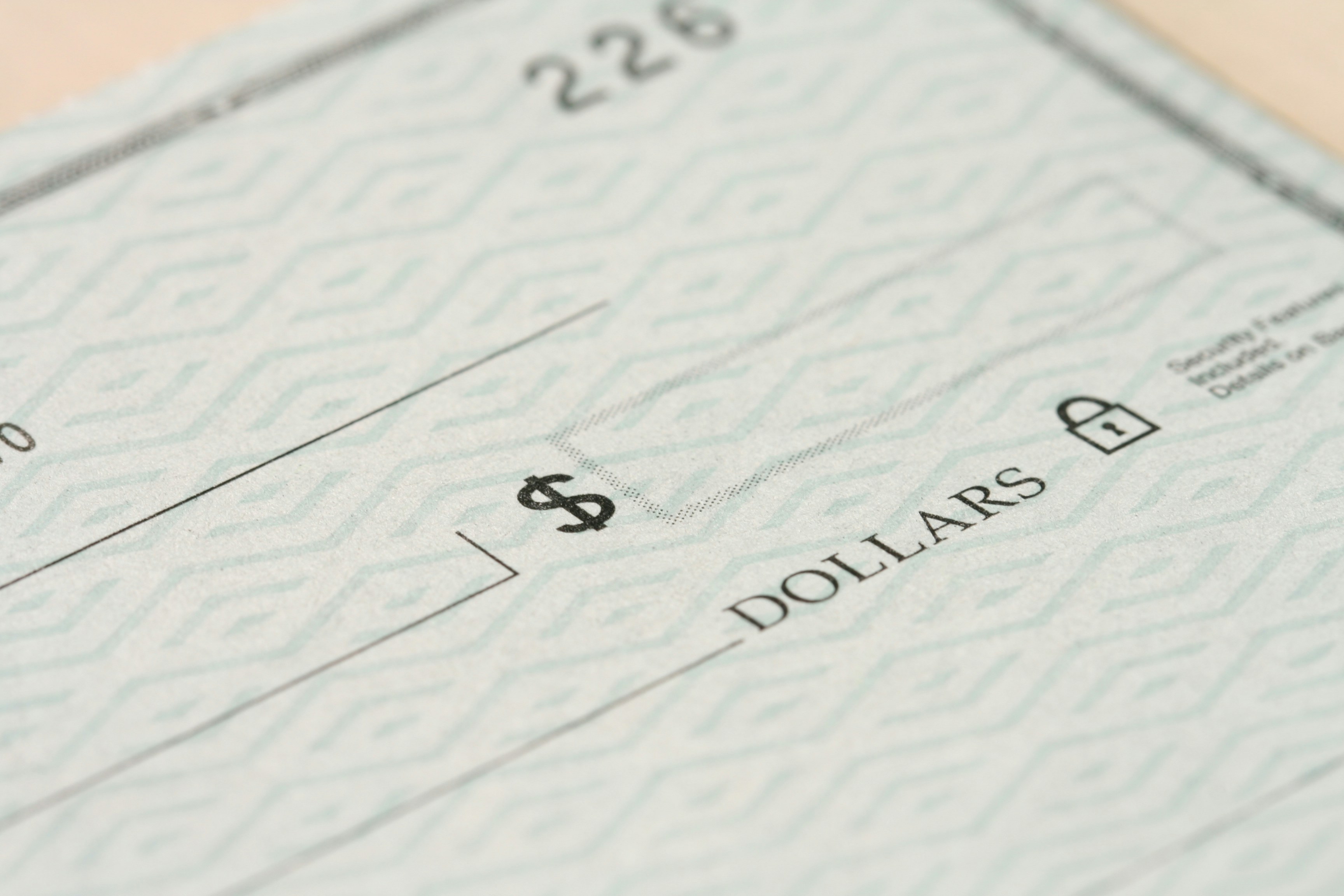
This blog post discusses the ongoing issue of check fraud in 2025, highlighting its impact on financial institutions and customers, and explores how technology-driven solutions like AI and automation can help prevent it.