In June 2020, Visa announced the launch of a digital tool that would help the US financial services institutions with their efforts to counter new account fraud. According to Visa, new account frauds are estimated to be around $10 billion a year. So what is that tool, and why is it needed? This article explores what is new from the AI and ML desk to help FIs in fraud detection and prevention.
An eye-watering amount of money is being lost to fraud by individuals and companies every year. In 2018 alone, payment-processing companies sustained losses worldwide that amounted to $27.85 billion due to card fraud, with the figure projected to hit $40 billion by 2023. The challenging thing with financial fraud is that most of them go unreported, primarily due to the fear of losing customer confidence, making projections like these reasonably conservative. As a result, banks and other financial institutions (FIs) have deployed various tools to detect and prevent fraud.
In the past, such tools included an army of analysts who would try to keep up to date with emerging fraud techniques to identify and flag suspected fraudulent activities. This was a cat-and-mouse (often hit-or-miss) scenario, which ended up being more like mitigation and recovery efforts after the fraud happened than a preventive strategy. But now, financial institutions, from banks to payment processors, use artificial intelligence (AI) and machine learning (ML) to help prevent fraud. It is data-driven and a more robust alternative to the fatigable human analysis of fraud. This is because the customers are now churning mountains of data to which the old, rule-based approach to analysis can no longer be applied.
AI and ML in Identity Management
Identity theft is one of the most common forms of fraud. This is because banks and other financial services companies used to rely on government-issued forms of identification as the only reliable way to identify their customers. Fraudsters have been able to circumvent this kind of identity check by producing near-perfect identification documents. And because there are no standardized security features that cut across all these forms of identification, it is far more difficult for an institution to deal with a customer from a different territory.
AI- and ML-based identification authentication services come in handy when using other forms of unique identification, including biometrics, to ensure the integrity of customer identification data.
These services help banks and financial services companies easily and confidently onboard customers regardless of their geographical location.
AI and ML in Transaction Monitoring
With banks and businesses being far from having the ability to identify real persons behind transactions confidently, fraud can occur at any stage, even after the customer is onboarded. AI and ML are used to analyze vast amounts of data to identify patterns in a customer transaction based on previously known data for the system to flag the transaction or allow it to proceed autonomously. One weakness of the human-based approach is the inevitability of preconceived bias. AI and ML systems have no such problems because they base their decisions purely on the available data. Such systems are used to continuously monitor customer behavior and make broad yet accurate correlations between such behavior and subsequent activities. It is one of the ways banks are able to flag certain accounts that do not comply with certain preset parameters, such as transaction limits, geographical location, and the medium of the transaction. Beyond these broad applications, AI and ML have more targeted uses, such as the following:
- Know-your-client (KYC) and anti–money-laundering (AML) applications: These are used to onboard customers and ensure that their banking activities conform to their declared sources of income to detect any variances and prevent money-laundering activities. AI and ML also help banking institutions continuously monitor sanction lists on a real-time basis.
- Internal controls: While fraud is usually viewed as an external problem, internally facilitated fraud is just as common. Bank employees are usually susceptible to social engineering or outright collaboration with fraudsters to gain internal access. AI- and ML-based tools can help by tracking the system behavior of employees in real time and flagging suspicious transactions.
- Loan and credit applications: AI and ML help in the analysis of customer data from non-traditional sources, including social media, to build an accurate credit profile and help in making informed lending decisions.
- Credit card fraud prevention: The most-used form of online payment and settlement is the one most prone to fraud.
- Data and information sharing: AI and ML enable banks to share and analyze information, especially fraud data, to ensure that they are prepared for attacks that have happened elsewhere, allowing them to stay ahead of the game. Due to the interconnectedness of FIs, sharing information is critical in fraud prevention because it serves as a learning opportunity for others in the industry.
Although it would be impossible to eliminate all forms of fraud, available data shows that AI- and ML-based approaches are far better at it than humans are. ML algorithms get better with time as the amount of data increases. Aside from fraud being a direct cost to banks and other FI players, it is also something that regulators have levied hefty fines for, especially when it is demonstrable that the FI in question was negligent or did not have preventive measures in place, to begin with. As technology gets better, these systems could be deployed at a lower cost and would have expanded uses beyond just fraud prevention as well.
To learn about Prove’s identity solutions and how to accelerate revenue while mitigating fraud, schedule a demo today.

Keep reading
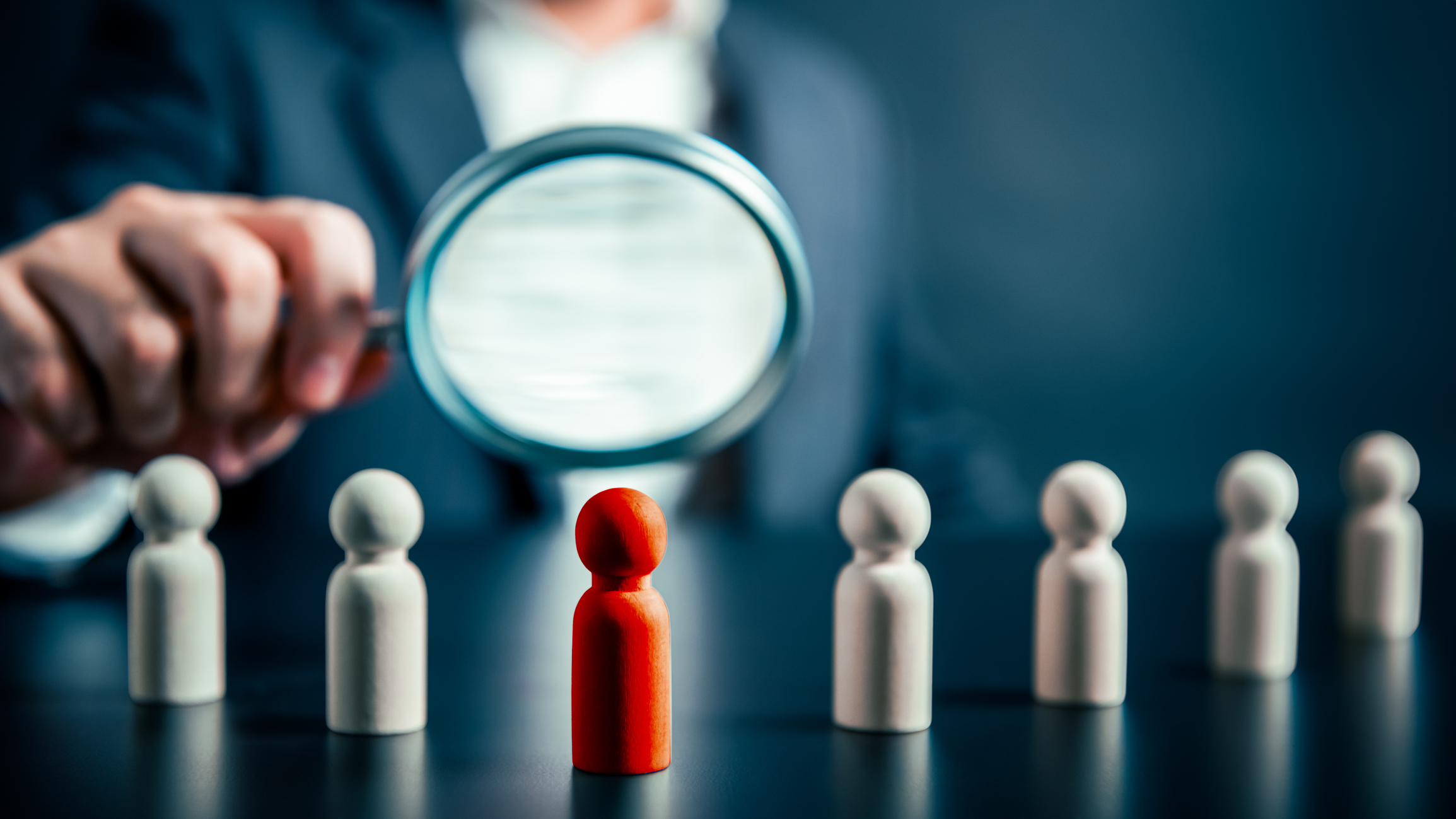
Discover the challenges of implementing financial fraud detection systems and learn solutions to stay ahead of evolving threats.

In this blog we break down the top six points to consider when it comes to choosing an identity verification solution.

Learn what check fraud is, how it works, and how banks are using innovative ways to eliminate check fraud activity.